Using Deep Research in Historical Work: Its Impact and Challenges
On 2 February 2025, OpenAI released a new tool called Deep Research. The tool is designed to help users quickly analyze huge amounts of data, identify connections between sources, create summaries, and suggest new directions for research on various topics.
It will also undoubtedly impact historians' work by speeding up the search for sources, identifying patterns, analyzing texts, and collaborating on complex projects. The tool doesn’t just speed up research; it opens up new avenues for historical discovery and interpretation.
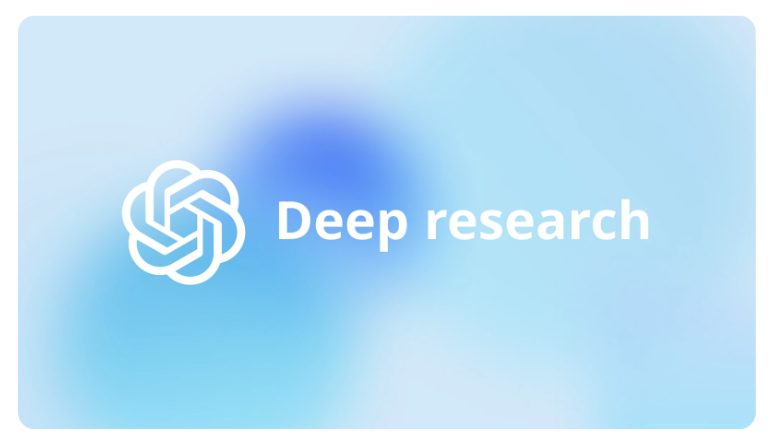
Advancements of Deep Research
Recently, Humanity’s Last Exam—a global benchmark created by nearly 1,000 experts from over 500 institutions across 50 countries—conducted a rigorous comparison of AI tools, evaluating their reasoning and subject expertise across more than 100 disciplines.
In this challenging 3,000-question expert-level test, the model powering Deep Research set a new record for accuracy at 26.6%.
Beyond the numbers, it stood out for its human-like reasoning—actively seeking out and integrating specialized knowledge rather than relying on rote memory.
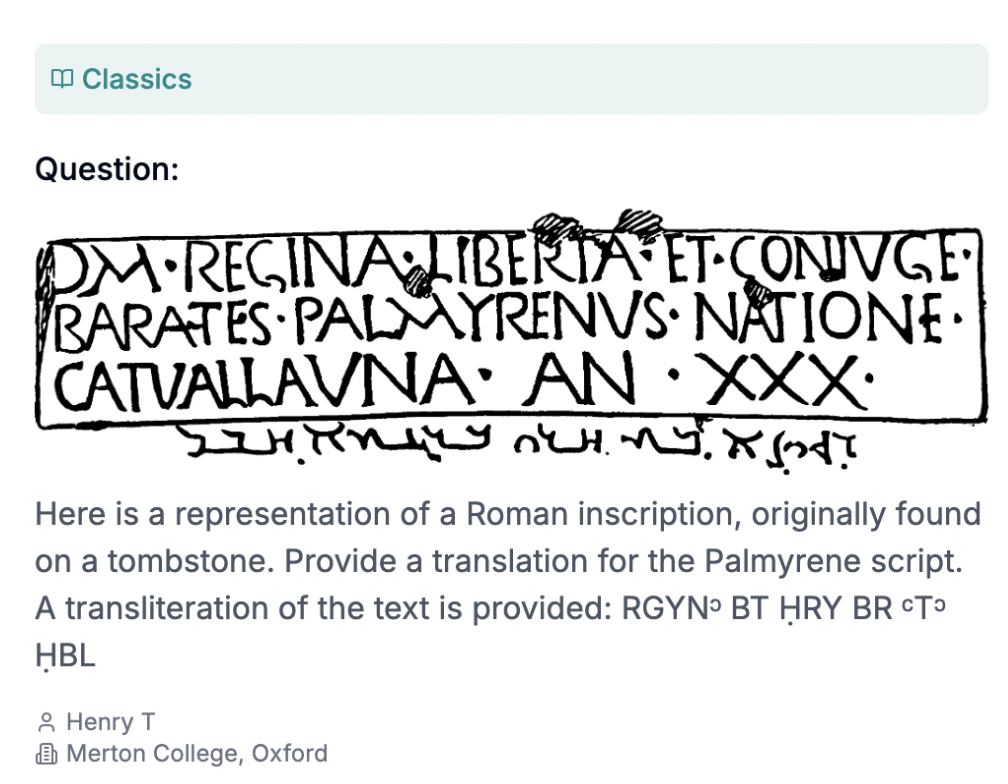
Using Deep Research to Explore Historical Contexts
In “I just tried ChatGPT deep research to dive into my family history — here’s what happened”, Amanda Caswell explored how ChatGPT's deep research can benefit users outside of academic and professional work. She tested it on her family genealogy to see how the average person could benefit from a more thorough exploration than typical web searches.
To get the best results, she advises gathering key family details like names, dates, and regions (there is no need for addresses). The more precise your data and queries are, the better the outcome. Rather than asking basic questions focused on facts or dates, deep research tools enable historians to address more complex, interpretive questions.
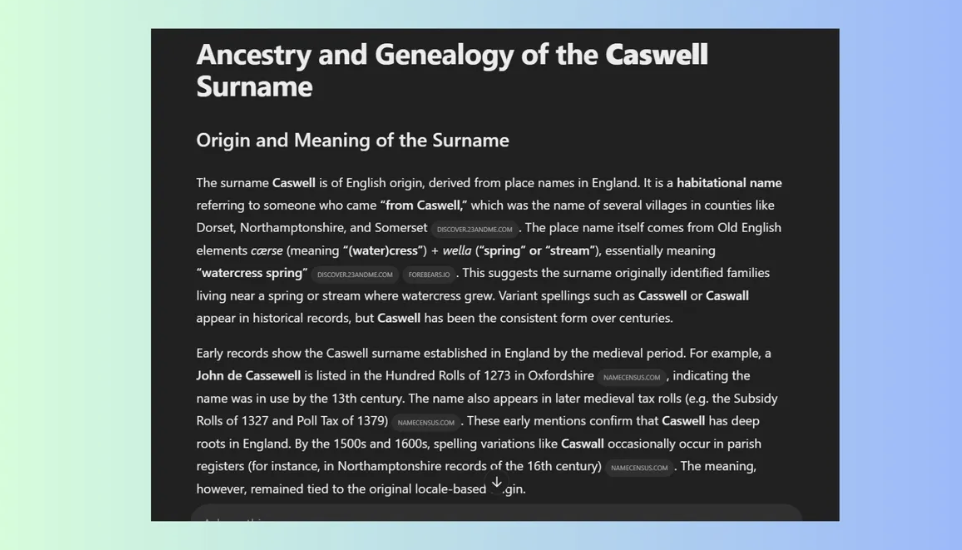
Deep Research Challenges and Limitations
Despite its advanced capabilities, Deep Research faces several challenges. The model may be susceptible to malicious inputs that could influence its outputs. Like many AI models, Deep Research can exhibit biases or generate inaccurate information "hallucinations".
Nathan Lambert’s “Deep Research, Information vs. Understanding, and the Nature of Science” explores the evolving role of AI tools like OpenAI’s Deep Research in scientific discovery. Lambert argues that while AI can greatly accelerate the processing and synthesis of information, acting as a powerful “engine of understanding,” it currently lacks the ability to independently generate new scientific insights. He emphasizes that AI excels at organizing and synthesizing existing knowledge, but does not replicate the human ability to make groundbreaking discoveries.
In “Is This the Last Generation of Historians?” Mark Humphries raises several questions about the work of Deep Research. His initial attempt was to commission Deep Research to undertake a historiographical analysis of the evolution of fur trade scholarship, in particular to compare and contrast Canadian and American academic approaches. However, since most of the sources are freely available and there is no paywall, the resulting bibliography is somewhat limited in scope.
The author also asked him to find Alexander Henry’s unpublished letters, a complex task that requires navigating outdated archival sites and searching catalogues. Deep Research independently combed the LAC catalogue and 21 other archives, including ArchiveGrid, producing a robust list that closely matched his own earlier findings. Not perfect, but on par with what a research assistant could produce—something unthinkable just a few years ago.
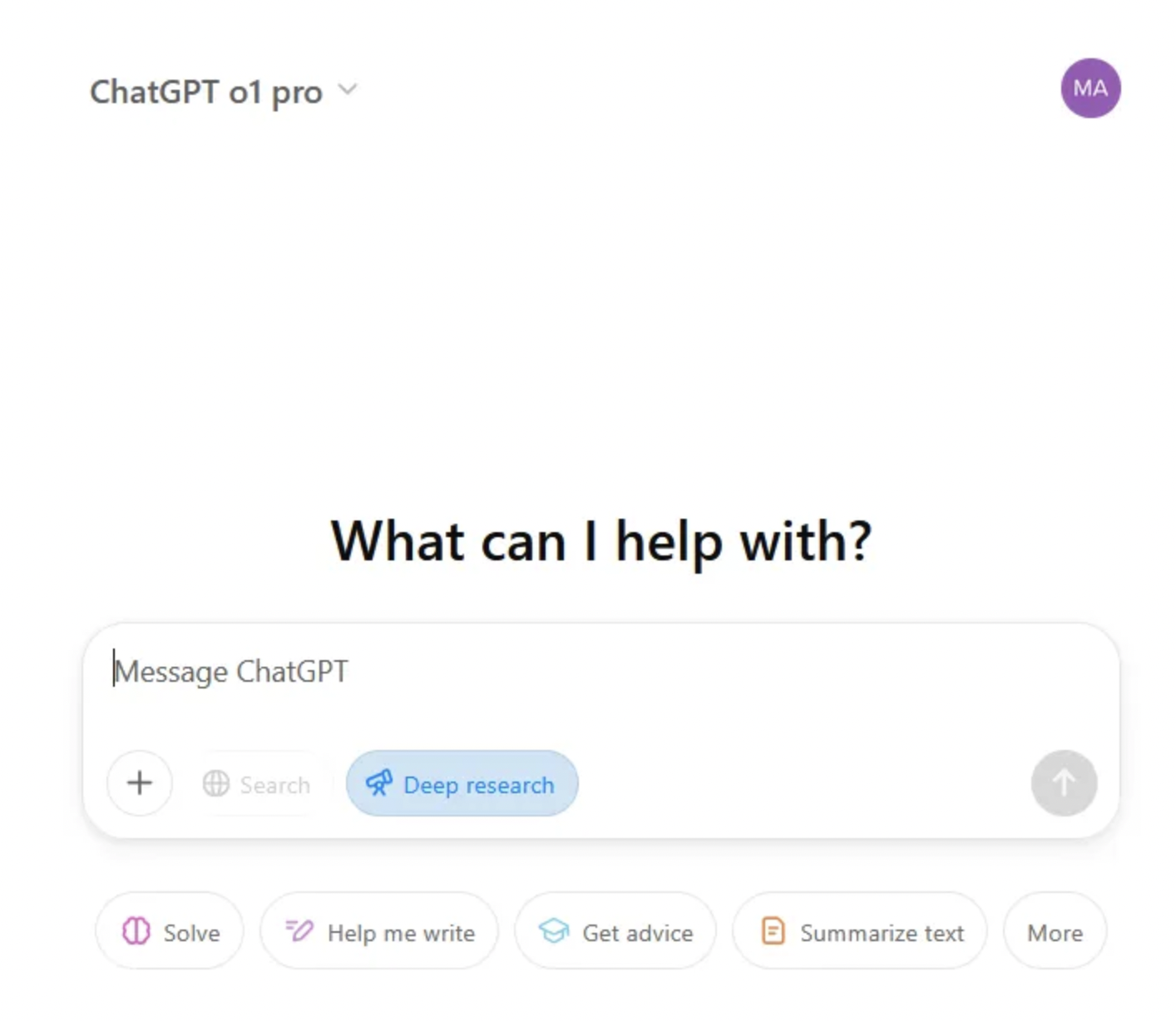
Deep Research has its limitations. Since it operates autonomously, a poorly worded prompt or misleading follow-up can send it off track without a way to correct itself.
In one of Mark Humphries’s tests, the model briefly descended into gibberish before quickly regaining coherence. Its most intriguing output came when asked to compare Alexander Henry’s 1809 Travels with earlier travelogues. Deep Research returned a 6,165-word analysis, claiming to use computational methods and close reading to identify stylistic parallels.
However, the code provided didn’t match its results, and many quotes were fabricated or misattributed. This suggests the model may simulate capabilities it doesn’t fully possess, possibly due to a lack of access to actual texts.When asked for a qualitative analysis without code, however, the model was more accurate.
Conclusion
AI now can address complex research challenges with unprecedented speed, posing a direct challenge to traditional academic methodologies. Historians, in particular, stand to benefit from these tools, using them to investigate detailed questions and uncover new insights.
However, the ongoing issue of hallucinations—especially when dealing with historical facts—remains a significant concern. While debates surrounding ethics and quality persist, the most pressing challenge is ensuring the accuracy and reliability of AI-generated research in the context of historical scholarship.